Not Your Grandmother’s Technology
Radar is over a century old and was first used in 1904 by Christian Huelsmeyer to detect ships. Well known applications are military radars, civil air traffic control, and, of course, speed traps for personally owned vehicles. But there is a misconception today that the technology is mature and few developments are happening in the space. New game-changing innovations are occurring in both imaging radar and cooperative radar. How Analog Devices, Inc. (ADI) is implementing radar and bringing unique software and algorithms to the application in the automotive space is what’s special.
For over a quarter of a century, ADI has been active in automotive, servicing both passive and active safety applications. For the past 15 years, ADI has been present in the supply chain for automotive radar with its DSPs and data converters, as well as more recently with its 24 GHz and 77 GHz/79 GHz radar chipsets.
“Advanced driver assistance systems are here, vehicle autonomy is coming, and road safety is paramount. So, what drives my work, and what drives our engineers, is using the most advanced capabilities and technologies available to realize higher performance and higher levels of autonomy to save lives,” said Chris Jacobs, vice president of autonomous transportation and safety at ADI. “Automotive sensors based on our products, by our estimate, save eight lives each day.”
Much work is required in both hardware and software innovation to ensure drivers, passengers, and pedestrians are protected. A more efficient and optimized radar technology must be developed, delivering the same high performance, functionality, and reliability of systems created for the aerospace and defense industry—transferring it to a form factor and cost appropriate to the personally owned vehicle market.
Mike Keaveney, technology director for the autonomous transportation and automotive safety business unit at ADI, said, “While the cost of a $250,000 high resolution imaging radar system is a mere pittance to the overall price of a multimillion dollar military tank, it’s grossly misaligned to your average $30,000 family car. We’re exploring tailoring it—miniaturizing it, ruggedizing it, and bringing down the cost, size, weight, and power requirements so it can be found in every automobile on the planet.”
Radar’s Challenges
There are significant technical, aesthetic, and economic challenges to transferring and adopting military and aerospace’s high cost, high performance radar technology and fitting it in a car. The key challenge is not only to reduce size, weight, and power (SWaP), but to improve performance while holding costs down. Radar must graduate from not only object detection, but object classification. This requires much more resolution in the radar image than is available in today’s best-in-class systems.
These are the goals ADI has set to advance the technology, ensure safety, and bring efficient and cost-effective automobile radar to consumers.
Performance
- Increase the angular resolution to the levels required for highly automated driving without blowing up the size, cost, and power consumption budgets.
- Increase the number of reflection points from low reflectivity targets.
- Dramatically reduce the latency of detection, particularly for laterally moving objects, which improves response time and allows vehicles to take evasive action in an emergency.
SWaP
- Optimize the form factor (size, weight, and power) while maintaining a high level of performance.
- Maintain the aesthetics of the system without compromising the industrial design of the vehicle.
Cost/Economics
- Implement high resolution radar at a price and form factor acceptable to mass market automotive cost constraints.
- Work within the car buyers’ price sensitivity range—they are the ones paying for it all.
Regulatory
- Continued compliance on government regulated advanced driver assistance systems (ADAS) safety features (such as the 2022 U.S. automatic emergency braking mandate) must also be maintained. Radar will no longer be an option; it will be standard. Therefore, it is critical to continually push system cost down to a price point consumers can absorb and OEMs can live with, but still maintain the performance needed for these challenging ADAS applications.
Smaller than the size of a cell phone, today’s automobile radar devices can detect if there is a large object in front of you, behind you, or in your blind spot off to the side. But that’s not enough.
Imaging Radar
The concept of imaging radar and achieving higher levels of angular resolution is a desirable capability, particularly for robotaxis. High resolution enables not just object detection (there’s something ahead) but object classification (there’s a bike, car, person, or child ahead).
To realize higher resolution, imaging radar utilizes high bandwidth signal processing along with digital beamforming and phased array techniques. All of this is massively hardware and processing power dependent where antenna size scales with the desired angular resolution and channel count increases to cover the required antenna area with channels. “Just throwing more costly hardware at the problem is a ‘brute force’ way to bring higher resolution as a solution,” said Chris Jacobs.
Today, ADI is working closely with leading OEMs and tier 1s on the development of new groundbreaking approaches to improve radar and address its modern challenges. Radar, as implemented in today’s automobiles, has a very coarse resolution. All it sees is a blob. It can detect something around a car—it could be a motorcycle, person or a large truck—but it cannot identify the object. By increasing resolution, via advances in hardware sensing technology and software algorithms, radar can discern various attributes around what it’s seeing—bringing us one step closer to a fully autonomous, safe vehicle.
The Resolution Issue and the Object Separation Challenge
Current conventional automotive radar offers a horizontal angle resolution of about 10° to 20° in a wide field of view.
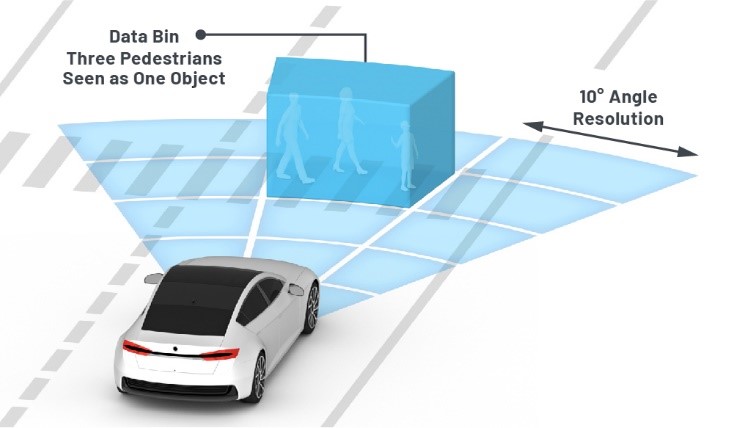
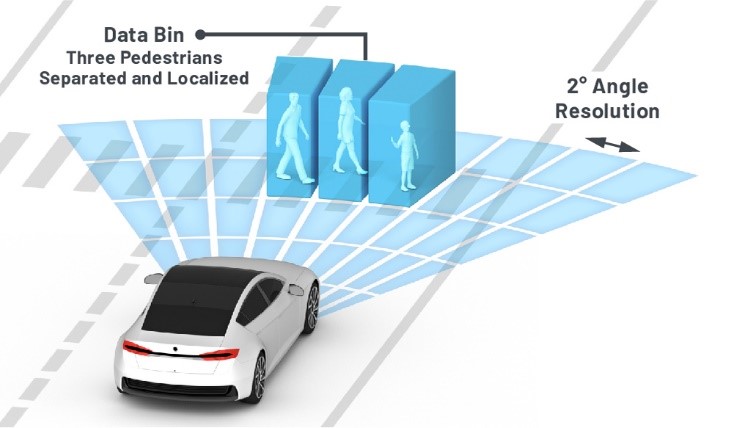
Imaging radar featuring 1° to 2° angle resolution—a 10× increase over non-imaging resolution. Data bins of information at 1° to 2° resolution separate and localize the three pedestrians.
Data Processing
The price you pay for more resolution is more data; as resolution increases, the volume of data goes up accordingly, resulting in the need for more computing power. That’s why an advanced schema to process all of the data efficiently is critical to manage a high volume of data along with low power consumption. Efficient central processing, or edge processing, will be fundamental to the future of radar.
The Next Step: Cooperative Radar and the Need to Communicate
“Cooperative radar, making use of existing radar sensor hardware in the car, is the way of the future in the automotive space,” said Mike Keaveney. “Cooperative radar is all about coherency and recognizing the need for things—in this case, sensing radars—to cooperatively create a high resolution coherent image. Once you can get cooperative radar to work economically, it has a number of advantages.”
Cooperative radar can provide imaging radar performance without significantly increasing the size of the individual existing radar systems in cars. This is because the effective aperture is now set by the distance between two (or more) distributed radar sensors with overlapping fields of view—not predetermined by the physical size of any one sensor.
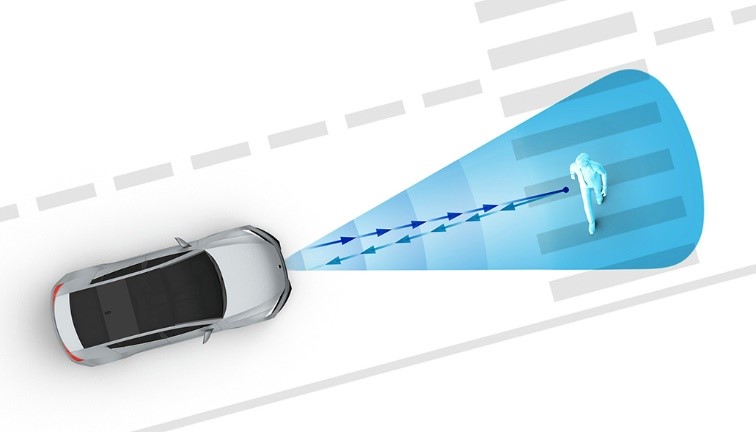
Primary radar is what is commonly used today in automobiles.
Radar transmission from each source bounces off an object and travels back to the point of origin. The aperture, or performance in primary radar, is in inches— the width of radar transmitter itself.
The Larger Aperture of Cooperative/SuperRADAR
SuperRADAR is ADI’s approach to achieve coherency algorithmically with multiple radar beams that have overlapping fields of view.
The SuperRADAR-based cooperative radar uses a low speed link for rough timing between radar sources. Each sensor sends data to the central processor or may send data from one radar to the other and do the processing at the edge sensor, which is a more economical approach.
“Traditional cooperative radar systems are not easily implemented given the need to run a high frequency link between radars,” said Chris Jacobs. “There is a very significant hardware-dependent overhead associated with achieving this type of coherency and substantial cost.”
There is a huge imperative to improve cooperative radar’s price over performance ratio for automotive radar. “The old ways of just adding hardware alone to the car is not the solution. We must bring new thinking to the problem,” said Jacobs. “There are intelligent ways in which we can algorithmically combine these technologies to increase the combined system performance with the same hardware in the system. ADI’s SuperRADAR approach allows the radar system to produce coherent superposition of multiple noncoherent images.”
Cooperative Radar
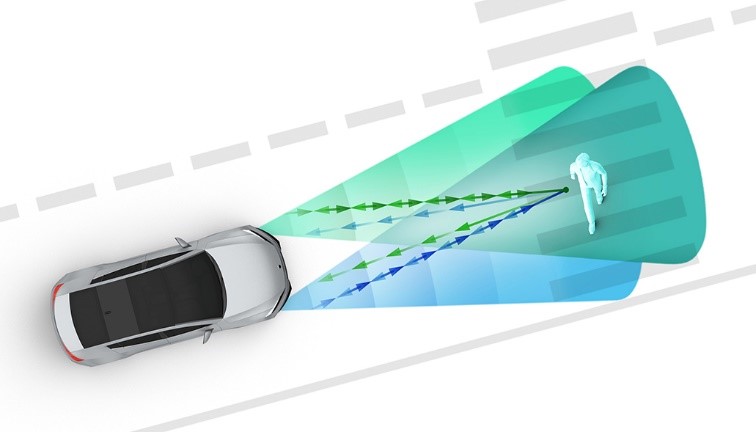
How does cooperative radar work? The transmission from each source bounces off an object and is captured by both radar receivers. As a result, you get 2× the look (or two different views) on the same target, and 2× the time on the target, as opposed to one look and 1× time with primary radar. Additionally, since both radars are operating cooperatively, the radar aperture (proportional to performance) is the size of the front of the car—the distance between the two corner radars (approximately 4 feet), as opposed to inches with primary radar.
This approach allows for cost-effective sensor designs that can be placed at multiple points around the vehicle to support superior object detection and classification.
The Benefits of SuperRADAR: 1 + 1 > 2
SuperRADAR not only reduces SWaP, but brings more capability to the system, achieving higher resolution, while using far less hardware, thus increasing application performance within a more reasonable cost envelope.
More Reflection Points: 2× the Time on the Target
SuperRADAR delivers twice the performance with the same amount of hardware. Alternatively, the approach can maintain the same performance using half the number of radar channels. Chris Jacobs said, “With SuperRADAR, we get twice the resolution that individual radar could achieve on its own. Additional processing power will be needed, but the roadmaps of automotive-grade DSPs/MCUs can adequately address these processing requirements.”
SuperRADAR is, in effect, radar fusion—we are fusing two independent radar views and, as a result, getting better resolution than what could be accomplished independently. Jacobs said, “Fusion will become the standard way of implementing ADAS in the future.”
Reduced Latency: Calculating Lateral Velocity, Quickly, Saves Lives
A key focus in vehicle imaging systems is the ability to quickly calculate lateral velocity—the speed of an object traveling orthogonally (at right angles) to the direction of a vehicle’s travel. However, even the best machine learning algorithms, largely based on cameras, require ~300 ms to make a lateral movement detection with sufficiently low false alarm rates. For a pedestrian walking in front of a vehicle traveling at 60 mph, milliseconds can make the difference between no injury and life-threatening injuries, so response time is critical.
The 300 ms delay is due to the time required to perform delta vector calculations from 10 successive video frames—the number needed for reliable detection with acceptably low false alarm rates. However, thanks to the wide effective aperture of SuperRADAR and the way it coherently combines the images from two or more sensors, it has the ability to accurately compute the tangential, as well as radial components of the velocity, in a single 30 ms measurement cycle (this latency is 10× faster than today’s best-in-class systems). This low latency of detection is below the 100 ms it takes a Formula 1 driver to react and well below the reaction time of a typical human driver!
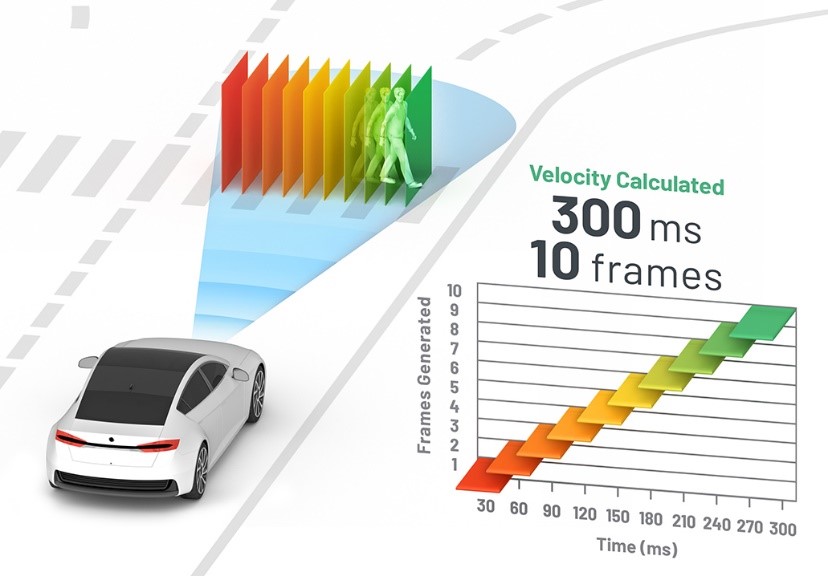
Using today’s common imaging radar technology, if someone crosses the street, multiple camera images are required to show something is moving. Each camera image takes 30 ms. Ten images take 300 ms. During that timeframe, the car moves several feet.
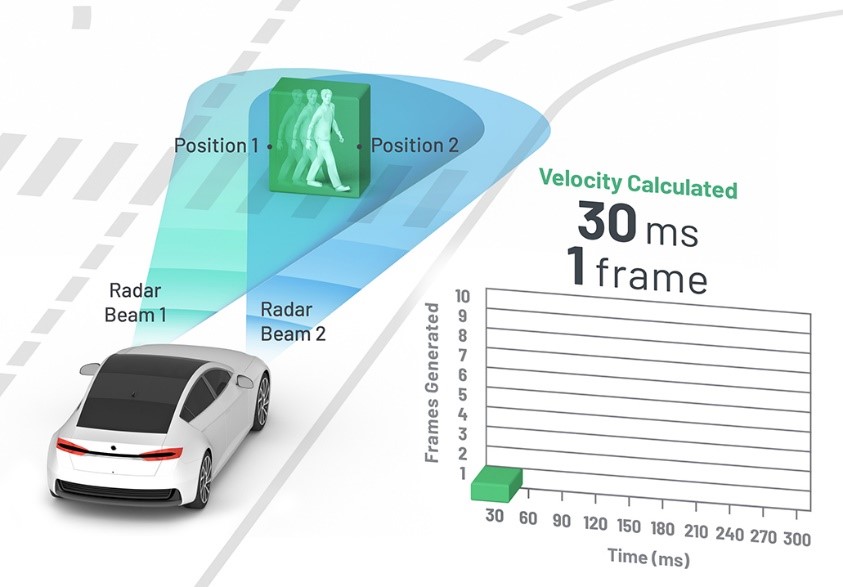
With two radars working cooperatively, triangulation can occur, capturing the object in motion because the two radar sources are offset. All that is required is to map the person first from Position 1 with Radar Beam 1, then, 30 ms later, from Position 2 with Radar Beam 2. This lets the automobile know where the person moves.
SuperRADAR enables the recognition of a moving object that crosses the path of travel in a one-tenth of the time of traditional imaging radar.
The Economics of SuperRADAR
The SuperRADAR concept is not only an efficient method for reducing overall system cost, but also hits performance requirements and brings more value to the end application.
Chris Jacobs said, “What you want is imaging radar performance, now only found in costly robotaxi applications, and to eliminate all the expensive hardware and bring the price down to something a personal vehicle owner can afford. This is where SuperRADAR comes to play, with a minimum hardware footprint and software that sits on top of it and to produce twice the performance.”
The Future of Automobiles
When we look at the future of automobiles, it will require a fundamental rearchtecture of current systems. What the vehicle platforms are going to look like tomorrow is much different than today.
With extensive experience and expertise across verticals, ADI is in a unique position to bring more value to the end applications through a combination of hardware and software offerings to optimize the radar processing needs in the cars of tomorrow. This algorithmic approach squarely addresses the total cost of ownership (TOCO) challenges facing auto makers today and into the future.
SuperRADAR offers numerous possibilities that have only begun to be explored. The technology is not only a higher performance, cost-effective solution advancing ADAS, but it will ultimately save lives.
About the Author
Chris Jacobs joined ADI in 1995. During his tenure, Chris has held a number of design engineering, design management, and business leadership positions in the consumer, communications, industrial, and automotive teams. He is currently the vice president of the autonomous transportation and automotive safety business unit at Analog Devices. Chris has a Bachelor of Science in computer engineering from Clarkson University, a Master of Science in electrical engineering from Northeastern University, and a Master of Business Administration from Boston College. He can be reached at [email protected].